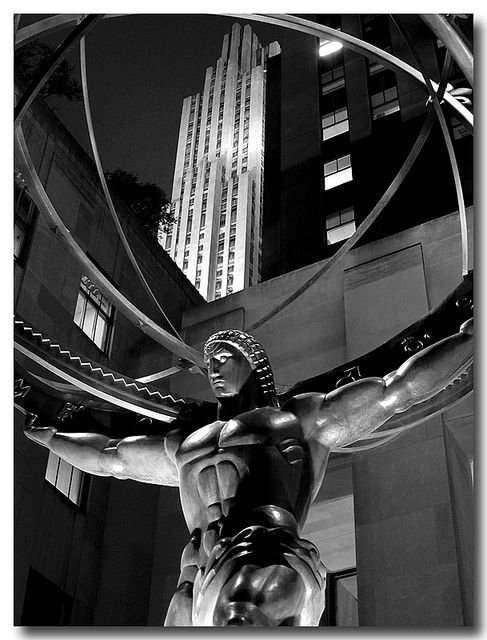
Neuro-Symbolic AI
RESEARCH
E n a b l i n g
SAFER AI
We are tackling some of the most pressing issues of current AI, holding back most of its great potential for societal and commercial value, much of which is in “cannot-fail” industries such as medicine, law, or finance:
Hallucinations
and other sources of non-factuality, including the unvettability of the Internet-scale datasets currently needed
Massive costs
for training current models, which create an effective oligopoly in AI and worsen worldwide energy issues
Unsafe behaviors
such as those of self-driving cars in new situations they haven’t seen directly in training
RECENT WORK
T h r o u g h
SMARTER AI
We focus on the foundational issues which are the root causes of today’s safety related issues. For true safety, AI systems must understand what they read (not just mimic sequences of text), what they see around them, and the implications of their actions; and they must be designed with humans in mind.
Understanding
Reasoning
Neural networks still do not reason reliably. We showed conditions under which a broad new class of reasoning systems, including forms underlying some neural networks, can be confirmed to reason correctly, in Fagin et al., Proceedings of the National Academy of Sciences, 2024.
Semantics
AI models still do not explictly represent meaning, or semantics — the basis needed for a true “world model”. In 1952, a seminal proposal to add meaning to information theory, one of the pillars of AI, was put forth but was never developed mathematically enough to be usable. We showed the first theoretical foundations unlocking the potential of semantic information theory, in Lastras et al, arXiv 2024., to be submitted to journal.
Artificial general intelligence (AGI)
Compositional generalization
Reliable out-of-distribution (OOD) behavior (i.e. handling new situations that were not seen in training, not just mimicking already-seen solutions) and the potential to learn with dramatically less data are widely understood to require “compositional generalization”, but this notion in AI has remained mostly anecdotal. We provide firm definitions and foundations in Ram et al, International Joint Conference on Artificial Intelligence, 2024.
Causality
The ability to reason correctly about cause-and-effect (vs correlation) is crucial for AI systems, yet existing theory still only holds for certain idealized settings. We began exploring how certain probabilistic semantics can allow modeling of causality in more realistic partially identified and cyclic cases, in Cozman et al., International Journal of Approximate Reasoning, 2024.
Human in the loop
Interpretability
In general, AI models that perform well are difficult for humans to interpret, and vice versa. We show a model class that can achieve both state-of-the-art classification performance yet achieves a high level of interpretability, in Carrow et al., American Association for Artificial Intelligence, 2024.
Confidence
AI systems typically do not report their confidence in their answers, which can be misleading at best, and dangerous at worst. We continue development of a framework for probabilistic reasoning which propagates error bars on its inputs to error bars on its outputs rigorously yet efficiently, in Marinescu et al, Neural Information Processing Systems, 2024.
Our Research
PHILOSOPHY
Deep and long-term programs
Most of mainstream resarch consists of incremental techniques within existing frameworks, for many reasons including the incentive structures in existing institutions (big tech companies, government-funded research in academia). While this of course represents tremendous aggregated value, we created this organization to enable those who want to join us in exploring longer-term roads with transformative potential. While we will inevitably do some incremental work as part of intermediate progress, it is not our goal to do the common rapid-fire publishing of low-hanging fruit and 'minimum pubishable units' that will be completely forgotten 10 or 50 years from now. In every project thread, whether we ultimately succeed or fail, we are attempting to swing for the fences.
Multi-disciplinary and multi-approach
We draw inspirations from many sources to attempt to unlock ourselves from whatever is the standard thinking around any particular topic. We're always looking for ideas from other fields that have not been fully exploited in AI. With dynamic teams and a scientific open mind to multiple approaches, we may even pursue two exactly opposite approaches at the same time.
We mainly pursue work in three categories:
1. New foundations
Here we are establishing new fundamental machinery to ultimately enable entire new classes of techniques downstream, often toward providing a basis for "no-fail" AI systems via rigorous guarantees and understanding of their behavior. Examples include our threads on multi-dimensional sentences to analyze larger classes of logical systems and extending information theory to include formal semantics.
2. New methodological frameworks, or model classes
Large language models are an example of what we mean by a methodological framework - one with several positives and negatives that helps to inspire us to explore new frameworks. Each framework that we explore can be thought of as attempting to simultaneously achieve a certain combination of our various aforementioned desiderata for AI systems (interpretability, rigorous reasoning, learning with less data, semantic representation, etc), since they tend to trade off with each other. Our aim is to ultimately arrive at frameworks that can achieve all of the desiderata at once. Examples include our threads on logical credal networks for fully representing ignorance in probabilistic reasoning, logically-based neural networks for interpretability without sacrificing predictive accuracy, and extensions of the robust logic framework for efficiently learning logical knowledge.
3. New solutions for important use cases
Across our various frameworks, we consider all of the most common AI tasks such as classification (e.g. as is common in data science), sequence-to-sequence transduction (e.g. as is done by LLMs), and sequential decision making (e.g. as is done by robots).
Though applied work is not the general focus of the Institute, we do pursue selected applied threads to make sure our techniques have the ultimate real-world applicability to no-fail use caes that are important in society. Examples include our work in medicine on doctor-interpretable models of wound progression. Our larger window on real-world needs is through our industry-facing sister organization.
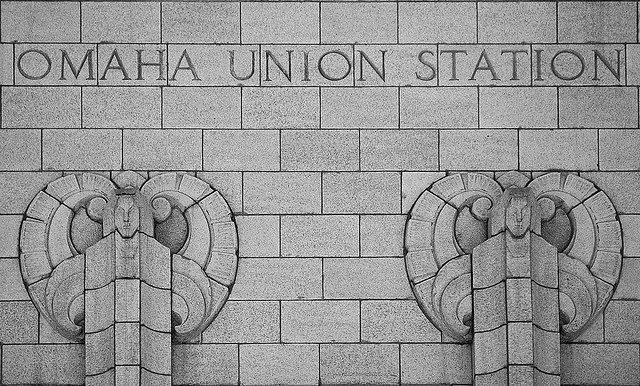